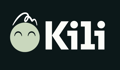
Best Practices In Collaboration & Project Management
The definitive guide for your data annotation projects
Download the whitepaper
High-quality training data is critical to delivering a successful AI-powered app.
But who says high-quality training data says... Data labeling, and likely: data labeling at scale.
To make the various stakeholders imply work together efficiently
But getting this high-quality training data is no picnic. Although necessary, the labeling process of raw unstructured is often perceived as a painful experience.
If any of these concerns ring a bell, this ebook on project management is for you!
By reading the end of this step-by-step guide, you will be familiar with the best practices companies can emulate.
More and more products are powered by machine learning. That’s why it's [capital] to think about ethics and to make sure [its] impact [is] positive.“
Clément Delangue, Co-founder & CTO
@Hugging Face
“Our Data-centric approach to AI has helped us achieve a categorization of our own categories that is accurate in more than ninety-five percents of the videos.”
Andrea Colonna,VP AI
@Jellysmack
“But the real-world experience of those who put them into production shows that (...) it's often the quality of data (...) that makes your AI project succeed or fail.”
Edouard D'Archimbaud, Co-founder & CTO @Kili Technology