Practical Tips and Solutions for Implementing RLHF
Reinforcement Learning from Human Feedback (RLHF) is the final ingredient that makes frontier language models so powerful. Unfortunately, due to its technical complexity, the technique has remained the preserve of large model builders. Now, new tooling is making the technology more accessible, allowing businesses to train smaller open models that outperform traditional fine-tuning methods and closed models for specific business tasks.
This webinar comprehensively explores RLHF, covering its fundamental principles, significance in AI development, and how it can be practically implemented by all businesses. We'll also dive deep into the operational challenges of creating RLHF datasets, including scale issues, human expertise, and quality control.
Key takeaways:
- The core principles behind RLHF and how it works
- The benefits of applying RLHF to your business use cases
- Real challenges and solutions in creating RLHF datasets
- Practical strategies for implementing RLHF in your projects
Whether you're looking to implement RLHF for the first time or optimize your existing workflows, this webinar is designed to provide valuable, actionable knowledge.
Get to know our speakers
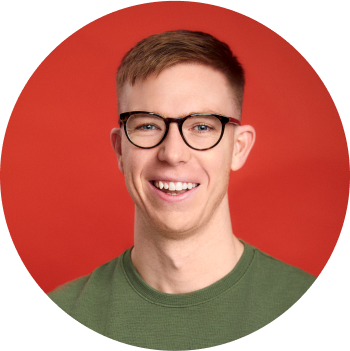
Andrew Jardine
Head of GTM
Adaptive ML
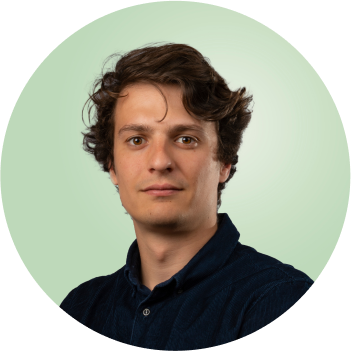
Paul Graffan
AI Alignment and Safety Expert
Kili Technology
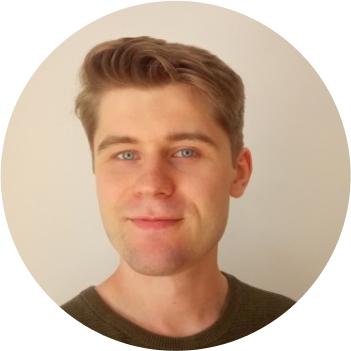
Daniel Hesslow
Cofounder & Research Scientist
Adaptive ML
Andrew Jardine
Paul Graffan
Daniel Hesslow
Kili Technology x Adaptive ML
Kili Technology
Today, LLM developers and fine-tuners are focused on unlocking the full potential of their frontier AI models. However, much of this potential remains untapped due to a shortage of high-quality data for training and fine-tuning LLMs. Additionally, there is a lack of quality human feedback to evaluate, align, and ensure the safety of these models. At Kili Technology, we address this challenge by providing custom, large-scale, high-quality datasets and human feedback. This helps machine learning engineers achieve their goal of delivering truly valuable models.
Adaptive ML
Unique Data for Frontier AI
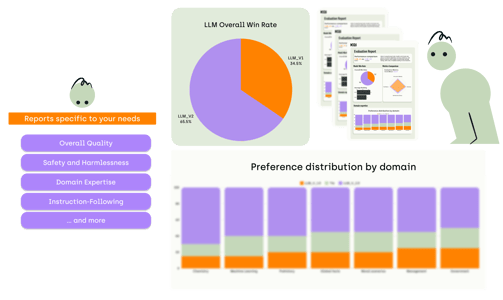